Let me tell you about Sarah, a marketing director at a mid-sized SaaS company. Every Monday morning, she’d brew an extra-large coffee and brace herself for the weekly grind: manually compiling competitor pricing data from 27 different websites, updating spreadsheets, and trying to spot trends in the chaos. This process consumed 15-20 hours of her team’s time every week – time that could have been spent on actual strategy and creative work.
Then everything changed when they implemented AI automation. What used to take nearly three full workdays now happens automatically before Sarah’s first coffee break. The system not only collects the data but flags pricing anomalies, tracks changes over time, and even suggests adjustments. Her team got back 60+ hours per month almost overnight.
This isn’t futuristic fantasy – it’s what’s happening right now in forward-thinking companies. And here’s the kicker: you don’t need a PhD in computer science or a seven-figure IT budget to make it work for you.
The New Workforce: How AI Automation Actually Works
Let’s peel back the curtain on how these tools operate in the real world. Traditional automation is like teaching a child to make a peanut butter sandwich – you have to specify every single step: “Pick up knife. Scoop peanut butter. Spread left to right.” Miss one instruction, and you get jelly on the ceiling.
AI automation is more like training a culinary apprentice. You show it several examples of what “done right” looks like, and it learns to handle variations – different bread types, spreading techniques, even making substitutions when you’re out of jelly.
The Three Pillars of Effective AI Automation:
- Intelligent Data Processing AI systems analyze unstructured data (emails, PDFs, web content) with contextual understanding—differentiating, for example, a numerical “price” in tables versus conceptual mentions in text. Practical applications include sentiment analysis of restaurant reviews to track dish popularity and customer satisfaction.
- Continuous Learning Through feedback loops, AI progressively improves accuracy—like a recruitment tool achieving 83% “culture fit” prediction accuracy after 100 hires, dramatically reducing manual screening time while boosting hire quality.
- Decision Intelligence From basic rule-based actions (e.g., abandoned cart emails) to advanced predictive analytics, AI enables proactive operations. E-commerce systems exemplify this, auto-adjusting inventory by analyzing 15+ variables like demand trends and supply delays to optimize stock proactively.
The Hidden Costs of “The Old Way”
Most teams dramatically underestimate what manual processes are actually costing them. It’s not just about salary hours.
Consider the opportunity cost: Every hour your team spends on data entry or repetitive tasks is an hour stolen from strategic planning, client engagement, or innovation—activities that actually drive growth. Then there’s the error tax: Manual work inevitably leads to mistakes, and research from Harvard Business Review shows that correcting these errors can consume an additional 20% of time, not to mention the damage to client trust or flawed decision-making. Perhaps most corrosive is the burnout factor: Monotonous work drains creativity and morale—one tech company’s internal study found a 47% higher turnover rate among employees stuck in repetitive tasks compared to those working on dynamic projects.
A 2023 study by McKinsey found knowledge workers waste 61% of their time on routine tasks that could be automated. That’s not just inefficient – it’s actively preventing your business from reaching its potential.
Real-World Transformations Across Industries
1. Financial Services Revolution
JPMorgan Chase’s COiN platform analyzes 12,000 commercial credit agreements in seconds – work that previously consumed 360,000 lawyer-hours annually. Their error rate dropped from 8% to near-zero.
2. Healthcare Breakthroughs
At Mayo Clinic, AI automation handles prior authorization requests, reducing processing time from 20 minutes to 90 seconds while improving accuracy. This freed up clinicians to spend 30% more time with patients.
3. Manufacturing Insights
A German auto parts manufacturer automated their supplier quality reports. What used to be a monthly 40-hour analysis now happens in real-time, catching defects 3x faster and saving €2.7 million annually in warranty claims.
Your Step-by-Step Implementation Guide
Phase 1: Process Audit (Week 1)
To begin streamlining your operations, start by thoroughly analyzing your team’s daily routines to pinpoint the most repetitive and time-consuming workflows. Look for prime automation candidates—tasks that follow predictable rules, demand significant manual effort, and require minimal creative thinking. These “low-hanging fruit” opportunities often yield the quickest returns with the least disruption.
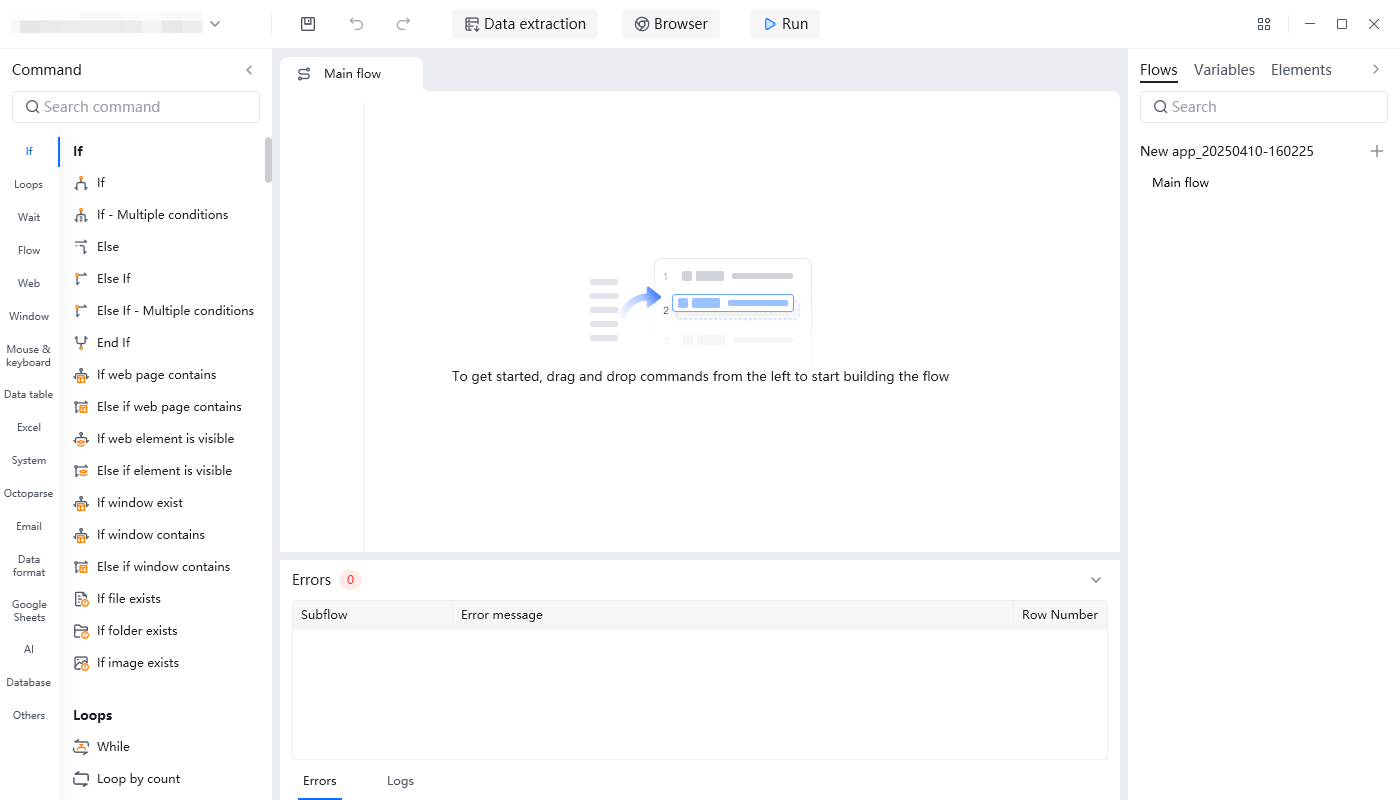
For deeper insights, consider observing employees as they work—pay close attention to moments of visible frustration or inefficiency. These pain points frequently reveal the best opportunities for automation, where technology can eliminate bottlenecks and free up your team’s time for higher-value work. A hands-on approach like this ensures you’re solving real problems rather than hypothetical ones.
Phase 2: Tool Selection (Week 2-3)
When it comes to data scraping solutions, different tools cater to varying skill levels and project complexities. Octoparse stands out as the most accessible option for non-technical users with its intuitive visual interface, while ParseHub offers more advanced features for handling intricate website structures. For developers seeking automated extraction, Diffbot provides a powerful API-based approach that delivers structured data with minimal configuration.
For process automation, teams have several robust platforms to consider. Octoparse AI combines user-friendliness with intelligent automation, making it ideal for beginners. Zapier remains a popular choice for its vast library of quick integrations across thousands of apps, while Make (formerly Integromat) offers deeper workflow customization for users who need granular control over their automations.
When evaluating these tools, four key factors should guide your decision:
- Ease of adoption – Balance the learning curve against the tool’s flexibility to ensure it matches your team’s technical proficiency.
- Integration capabilities – Prioritize solutions that seamlessly connect with your existing software ecosystem.
- Scalability – Choose a platform that can grow with your needs, handling increased data volumes or more complex workflows.
- Vendor support – Reliable customer service and documentation are crucial for troubleshooting and maximizing the tool’s potential.
Phase 3: Pilot Program (Week 4-6)
Begin your automation journey by selecting a low-risk, non-critical process as your pilot project. This allows your team to gain experience with AI tools without disrupting essential operations. The ideal candidate process should be rule-based, repetitive, and measurable.
Implement a parallel testing framework where both human operators and the AI system complete the same tasks independently. This side-by-side comparison provides concrete data on performance differences. Focus on tracking three key metrics: time efficiency (comparing completion speeds), accuracy (analyzing error rates), and output quality (assessing consistency and reliability).
Maintain detailed documentation throughout this trial period, recording setup processes, adjustments made, and performance outcomes. These records will form the foundation of your organizational automation playbook, serving as both a training resource and a blueprint for scaling successful implementations to more complex processes.
Phase 4: Scaling Up (More than 2 Months)
Successful automation requires equal focus on people and technology. Start with role-specific training—practical workshops for users, strategic overviews for leaders. Communicate changes transparently, emphasizing how automation eliminates drudgery, not jobs. Redesign roles to prioritize human skills like creativity and problem-solving.
Implement feedback channels for employees to report issues and suggest improvements, fostering engagement. After stabilizing the first rollout, expand to related processes using gathered insights. This measured approach builds confidence while developing staff capabilities. True transformation happens when AI enhances human potential, not just replaces tasks.
The goal is augmentation, not just automation—creating synergies between human and artificial intelligence.
The Psychology of Successful Adoption
Technology alone isn’t enough. Having guided 47 teams through automation adoption, I’ve found three human factors matter most:
- Control Comfort Start with low-risk processes, maintain human oversight initially, and gradually increase autonomy as confidence builds.
- Visible Impact Display time savings on dashboards, celebrate wins (“Saved 40 team hours this week!”), and share before/after comparisons.
- Growth Mindset Position automation as enabling strategic work, provide workflow management training, and create paths to higher-value roles.
When teams see automation as empowerment rather than threat, adoption accelerates. True transformation happens when technology serves people.
Future-Proofing Your Automation Strategy
The next wave of innovation is transforming how businesses leverage automation. Advanced conversational AI will interpret ambiguous requests, like locating “recent articles on automotive supply chain issues,” with human-like comprehension. Smarter predictive systems will proactively deliver needed outputs—such as auto-generating quarterly reports before meetings—by anticipating requirements.
We’re also entering an era of self-correcting automation, where systems independently identify and resolve operational errors without human intervention. Perhaps most crucially, ethical AI governance will become standard, with built-in frameworks for bias detection and regulatory compliance ensuring responsible deployment.
These advancements won’t just improve efficiency—they’ll fundamentally redefine human-machine collaboration, creating more intuitive, resilient, and trustworthy automated ecosystems.
Getting Started Today With Your Automation Journey
The biggest mistake I see? Analysis paralysis. You don’t need a perfect plan – you need to start. Here’s how:
- Pick One Process – Start small with something that hurts daily
- Try a No-Code Tool – Many offer free trials
- Measure Results – Even if it’s just time tracking
- Iterate – Build on what works
Remember Sarah from our opening story? Her team recently launched an innovative customer onboarding program – the kind of strategic project they never had time for before automation. That’s the real payoff.
Download Octoparse AI now to start your own automation processes!
The future belongs to teams who work smarter, not harder. Where will you automate first?
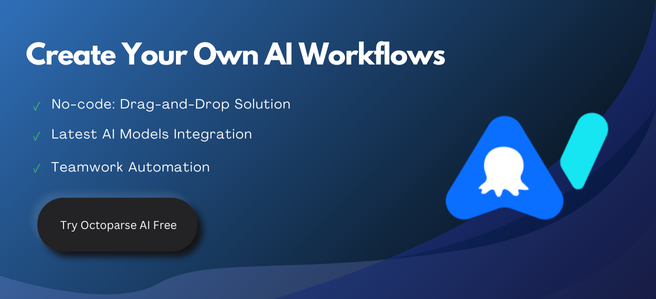